Itan lab is a part of The Charles Bronfman Institute for Personalized Medicine at Icahn School of Medicine at Mount Sinai. Our current research projects are listed below:
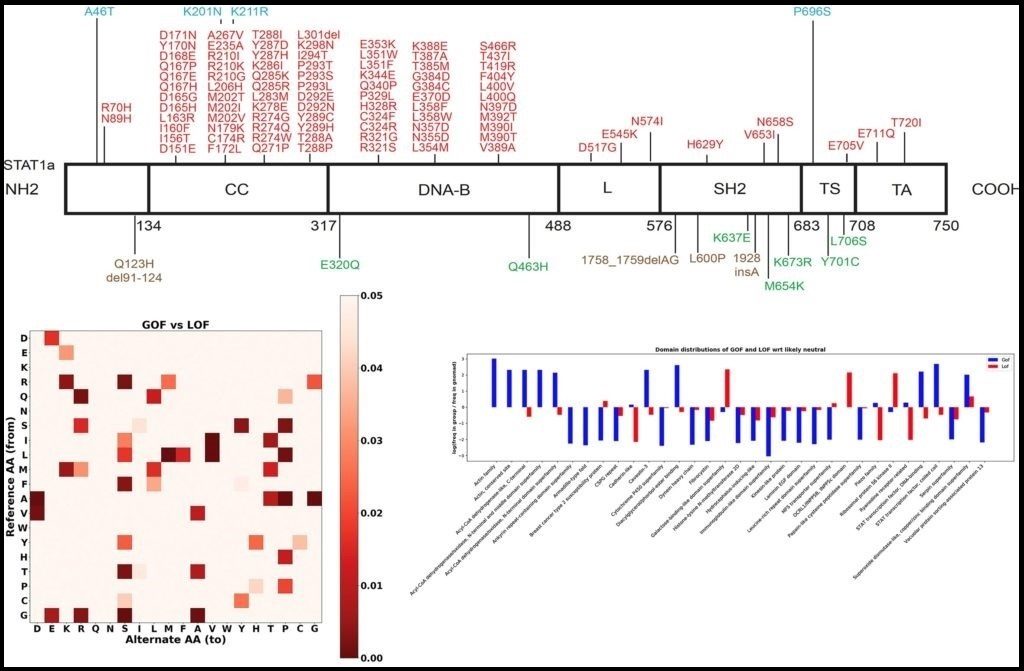
>> Predicting the functional consequence of mutations
Gain-of-function (GOF) and loss-of-function (LOF) mutations in the same gene result in different diseases and require different treatment. We aim to develop the first computational method to efficiently predict if a mutation is GOF, LOF or neutral by: (1) creating the first extensive GOF and LOF database by extracting data with natural language processing (NLP) algorithm on abstracts of known pathogenic mutations; (2) applying statistical and feature selection approach to detect protein-level and gene-level features that best differentiate GOF from LOF and neutral mutations; and (3) developing a Random Forest classifier and a public server to predict the functional consequence of mutations. We use Phenome-Wide Associations (PheWAS) on Mount Sinai’s BioMe resource for validating our resource and detect novel GOF/LOF phenotypes.
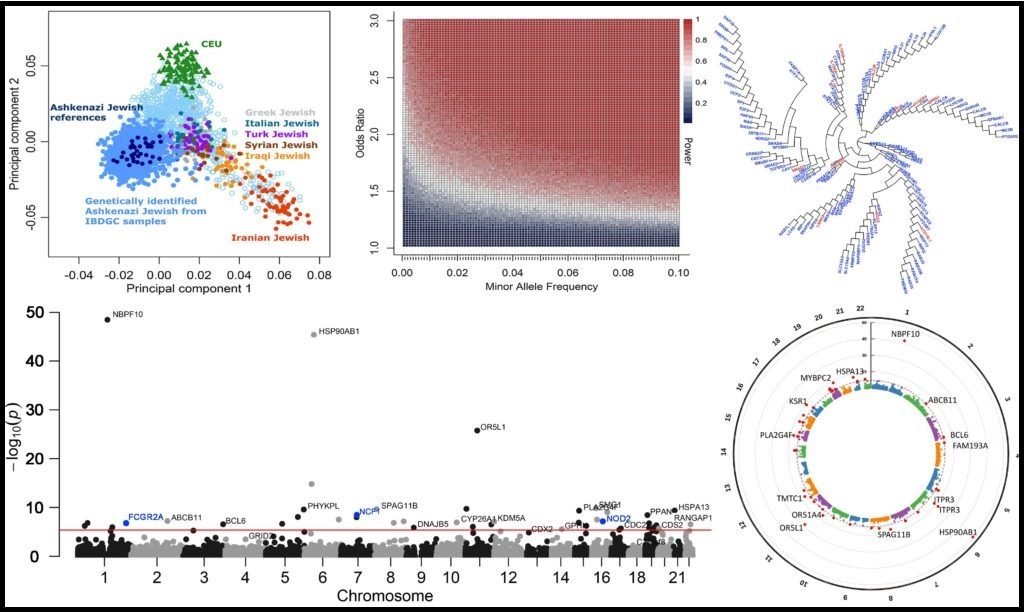
>> Investigating population-specific disease-causing mutations, genes and pathways
Different human populations display varying genomic architectures, that are likely to result in population-specific disease-causing mutations, genes and pathways. We currently investigate this concept with Ashkenazi Jewish (AJ) inflammatory bowel disease (IBD) patients from the IBD genetics consortium (IBDGC) whole exome sequencing data, that we identify by admixture and principal component analyses (PCA). We perform a gene burden analysis of cases vs controls, focusing on high-impact rare genetic variants. We use PheWAS to further validate our results. We aim to then extend the analysis to other human populations (Hispanic, African American and European) for identifying population-specific IBD genomic signals.
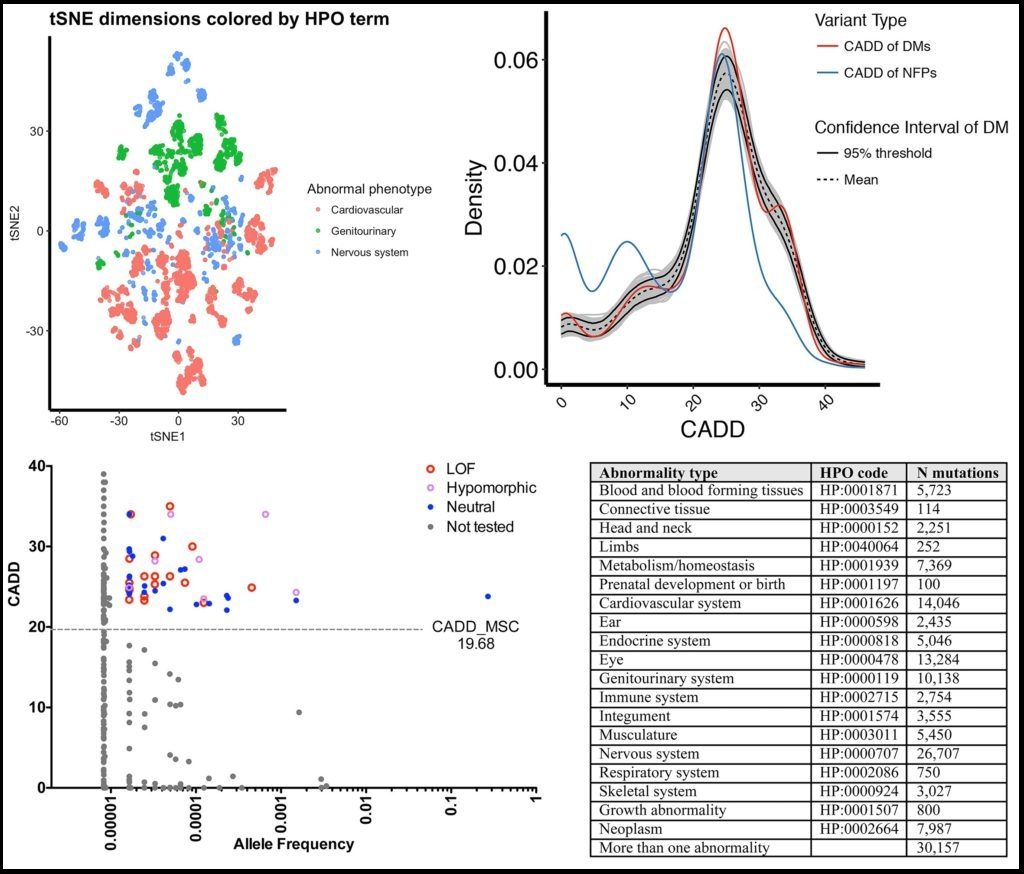
>> Deep neural network predictions of pathogenic mutations
While there has been an extensive effort in identifying pathogenic mutations in patients’ genomes, current methods still cannot efficiently prioritize the true pathogenic mutations in patients. We showed that by using extensive annotations it is possible to cluster mutations by disease groups. We aim to deep neural network (aka “deep learning”) classifier to efficiently and automatically prioritize pathogenic mutations in patients’ genomes, by considering the disease of the patient, train based on extensive annotations at the variant-, gene- and pathway-levels, and separate the training sets by disease groups and high-quality non-trivial neutral genetic variants.
>> Collaborative projects
GPCR mutations detection and investigation (with Judy Cho): using pattern recognition algorithms to extract GPCR mutations from the BioMe database, describing mutations by evolutionary conservation and opioid drug targeting.
Parkinson’s disease (PD) genomics (with Inga Peter): whole genome sequencing studies to identify pathogenic PD mutations and their overlap with IBD mutations.
Obesity genomics (with Ruth Loos): identifying novel obesity-associated mutations, genes and pathways by extensive biological annotations.
Disease genomics methods (with Jean-Laurent Casanova): developing novel methods to filter false positive variants, visualize human population genetic data, extract extensive DNA and protein sequences, and cluster pathogenic genes.
Protein features classification (with Avner Schlessinger): using machine learning methods to identify protein features that differentiate GOF and LOF mutations.
Primary immunodeficiency (PID) genomics (with Charlotte-Cunningham Rundles) extracting PID data from BioMe, annotating relevant exomes and identifying novel PID-causing mutations and genes.
Congenital heart disease (CHD) genomics (with Bruce Gelb): identifying novel de novo CHD-causing mutations and genes in an extensive trios’ cohort.
Brugada syndrome genomics (with Michael Gollob): gene burden and gene prioritization analyses for investigating Brugada syndrome genomics.